Abstract
This paper outlines a project to develop a software application tool – Smart Diet – for satisfying the meal-ordering requirements of people with cognitive and sensory disorders. Primary and secondary research were conducted to collect data, which was then analyzed using Paprika software. The meal design process was carried out using a free application version. It was selected for use because of its multiple flexibility and customization features, including the built-in dietary features that made it possible to customize the meal-ordering plan. The tool played a key role in creating an artefact that incorporates visual imagery features to improve the meal selection experience for patients with sensory and cognitive impairments.
This paper outlines the implementation plan, as well as benefits and potential limitations of integrating the new software into the healthcare environment. The impact of this analysis on the nursing profession lies in the capability of automated meal ordering systems to enhance patient care, particularly for individuals with special needs. Therefore, digital innovation is presented in this study as a creative tool for improving patient care in the nursing practice.
Introduction and Development of Artefact
Introduction
The quality of in-patient experiences within a health facility is partly affected by the eminence of meals provided (Ogata et al., 2019). Health outcomes are a function of the nutritional composition of meals served (Davis et al., 2022). Furthermore, hospital administrators have used paper-based meal-ordering systems to fulfill patients’ food order needs, as Diamond et al. (2022) outlined.
Mauch et al. (2018) suggest that advancements in Information Technology (IT) via automation have made it possible to improve meal-ordering plans. Consequently, computerized systems have made healthcare service providers more effective and efficient in meeting the needs of different groups of patients (Shukla et al., 2019). Broadly, these benefits have improved the quality of patient care in nursing.
Based on the reported benefits of computerized meal-ordering systems (CMOS), nurses have gained access to a unique tool for addressing the nutritional requirements of special needs patients (Yoon et al., 2022). As highlighted by MacKenzie-Shalders et al. (2020) and Bucci et al. (2019), even as patients enjoy the advantages of mechanized meal ordering systems, those with sensory and cognitive impairment disorders, such as dementia and autism, experience problems adapting to new digital systems because of their inability to operate smart devices or read menus on computer screen prompts.
Therefore, automated systems have failed to address unique population groups’ needs, undermining their overall efficacy (Hayes et al., 2021). Consequently, such systems need to be improved to make them holistic in addressing the needs of all groups of patients. The current project aims to develop a meal-ordering app for patients with sensory and cognitive disorders.
Rationale of Project
This project is intended to serve the needs of patients with sensory and cognitive disorders. Patients with dementia and autism fit the profile of these groups of patients (NIH Office of Communications, 2020). It is assumed that these patients experience challenges reading what is written on a menu (Koch et al., 2020; Zerón-Rugerio et al., 2019). Patients who have had a stroke or injury to the brain similarly form the same category of patients targeted for use in the new app.
The findings of the present project will be useful in improving the operations of the National Health Service (NHS), especially in in-patient care improvement (NHS-UK, 2022). The NHS is the largest publicly funded healthcare institution in the United Kingdom (UK) (NHS England, 2022a). Based on the nature of the organization’s operations, the findings of this study will be instrumental in improving patient care within the NHS structure (Ruiz-Mallorquí et al., 2021). NHS’s long-term strategy is designed to address the needs of patients with disability and those with learning impairments and disorders (NHS England, 2022b). Consequently, insights generated from the present project will contribute to realizing the organization’s vision of improving healthcare services for people with learning disabilities and disorders.
Artefact
Smart Diet is a meal ordering software that addresses the special needs of individuals with cognitive and sensory impairment disorders. It integrates visual imagery tools to aid special needs groups in enjoying automated meal ordering systems like other clusters of patients. Given that visual imaging tools have seldom been used to improve the usability of medical technology in meal ordering systems, patients with sensory and cognitive impairments continue to experience difficulties relying on readable texts to communicate meal requests (MacKenzie-Shalders et al., 2020). Thus, the Smart Diet artifact will help to address the unique needs of patients with cognitive and sensory impairments in meal planning and ordering (Rodríguez et al., 2018). Its success will be predicated on rationalizing meal-ordering systems to make them applicable to all groups of patients.
Literature Review
Search Strategy
The literature search strategy adopted for the current project was designed to understand factors that would affect the implementation of the smart app project. Data was obtained from credible data sources, including peer-reviewed journals and books. These materials were selected from four main databases: Emerald Insight, Sage Journals, Google Books, and Proquest. In adherence to the proposals of Harvey and Land (2019) and Alkhulaifi and Darkoh (2022), these materials were reviewed based on their relevance to the nursing field and patient care.
Additionally, research materials derived from these databases were selected based on their significance to the research issue and institutional vision of improving patient safety (Harvey & Land, 2019). In line with these requirements, the researcher ensured that the information gathered in the study was published within the last five years, 2018-2023. In processing the secondary research materials, the researcher assessed the abstracts, full texts, and state of journals, which discussed the research data.
Research materials were derived from the implementation of the research process using the following keywords: “technology adoption,” “meal ordering,” “healthcare,” and “patients’ experiences.” In this probe, 43 articles met the inclusion criteria, but 18 were excluded because their publication dates were outside the 5-year desired range. Some articles sampled in this review used empirical data to develop their findings, while others were systematic reviews of past research articles.
Review of Literature
Researchers have explored factors that ought to be considered when introducing new systems in the healthcare setting. For example, Ribbink et al. (2021) conducted one such study by sampling the views of 152 participants using the quantitative method. The researchers similarly collected views from 12 healthcare service professionals using the qualitative technique. They found that patients, especially the elderly, adopt alternative integrated systems positively. Using the mixed method approach was one of the study’s main strengths, but its failure to link findings with health outcomes and readmission rates made it difficult to quantify its impact on the broader healthcare system. Broadly, the findings point to various patient groups’ positive adoption of the Smart Diet software.
The optimism described above was shared by Vela et al. (2022) when they investigated factors that would lead to increased service satisfaction among patients who have suffered a stroke. This review was important to the present project because the proposed Smart Diet app is designed to appeal to patients with cognitive or sensory disorders. The investigation by Vela et al. (2022) sampled the views of 92 patients enrolled in a return home program using surveys. The study’s main strength is that the patients’ views were compared with central healthcare records to detect areas of consistency or divergence. Its main drawback is the lack of diversity among respondents and the small sample used.
A similar approach was adopted by Abhari et al. (2022) when they investigated factors that influenced the adoption of smart software in restaurants. After sampling the views of 150 respondents using the quantitative survey technique, it was established that perceived enjoyment and security were the two main drivers of technology acceptability (Abhari et al., 2022). The main strength of this finding is its large sample size, but its weakness is its focus on hospitality instead of the healthcare sector.
These findings are similar to those of Sensmeier (2021) above, which suggested that IT-enabled medical practices were useful in supporting heart-related medical processes, including those that treat atrial fibrillation and myocardial infarction. The main technological tools mentioned in both investigations included wearable devices, smartphone applications, and hand-held devices. The findings of both studies indicated that technological tools provided healthcare providers with important information regarding their health and well-being.
Sittig et al. (2020) argued that trends associated with software adoption included the development of digital tools for enhancing patients’ experiences and the progression of standard user interface designs that all groups of patients can accept. The researchers sampled 16 people’s views using interviews to develop the findings. This small sample size was the study’s limitation. However, its adoption of both qualitative and quantitative data helped bolster the findings’ reliability. Broadly, its linked findings ascertain the need to change the attitudes of nurses towards the adoption of new technology as a basis for implementing new ideas in the healthcare setting.
An article by Odendaal et al. (2020) used interviews and surveys to evaluate the role of employee attitudes in adopting new healthcare systems. Their findings indicated that the attitude of healthcare workers towards the adoption of new technology was responsible for its failure in the nursing setting. This project sampled the perceptions of 198 healthcare workers towards adopting mobile health technologies in the delivery of primary healthcare services. The study’s main strength was the large sample size.
Comparatively, Boehm et al. (2021) used a mixed methods technique to conduct a similar investigation. They reviewed 32 opinions of healthcare staff using the quantitative method and raised several issues in service planning, including creating a critical mass of personnel that would embrace and understand a new system. The small sample size was a limitation of the study because it meant that the findings could not be generalized. However, its focus on the healthcare setting means that its findings would be applicable in identifying challenges in technological adoption.
Alternatively, Mansfield et al. (2021) used the qualitative descriptive study design to identify issues hospital managers likely experience when introducing a new operational system in the healthcare setting. By interviewing 24 healthcare professionals, the researchers established that such changes would likely affect workflow systems, patient-healthcare service provider relationships, and team productivity (Mansfield et al., 2021). The small sample size was one of the study’s main weaknesses since this implied that its findings could not be generalized. These assessment areas are likely to be impacted by the introduction of the proposed Smart Diet application.
McPherson et al. (2020) analyzed the views of 15 healthcare service professionals to come up with similar findings. They opined that the lack of awareness regarding individual patient journeys was a source of operational failure for healthcare facilities that intended to introduce new systems without consultation. Therefore, a myriad of issues impact the adoption of new systems in the healthcare setting that could affect patient care. The main weakness of their investigation was the small sample size, but their use of the mixed methods framework was a strength because it helped compare different sets of findings.
Comparatively, Paoli et al. (2019) conducted interviews to investigate the same topic area. They cautioned that developing the highest quality Apps is a difficult task due to the multiplicity of factors involved in realizing optimum results. These views were developed after sampling the opinions of 17 healthcare staff. The small sample size was the study’s primary weakness. However, it advocated for the growth and development of processes and systems that may be executed at individual or institutional scales to improve health outcomes. In line with the findings of this article, the authors suggested that healthcare institutions have adopted new meal-ordering systems with varied levels of success.
As patients are always searching for personalized experiences, Gualandi et al. (2019) similarly used interviews to develop their findings after tracking the progress of orthopedic patients in a 250-bed health facility. Cumulatively, 13 respondents and their healthcare service providers were interviewed by the researcher to obtain data. Their findings were reliable, revealing that meal ordering was a new area of application that could expand the scope of technology use in the healthcare sector. They further argued that this practice is part of a wider professional engagement to improve patient satisfaction in the nursing care environment. One of the ways through which healthcare facilities have tried to meet these expectations is by customizing and expediting patients’ meal-ordering experiences.
Shukla et al. (2019) conducted a similar investigation involving 15 pre-diabetic participants to assess the impact of food order on their health outcomes. Their investigation was reliable because it took three months to complete, and the findings suggested that enabling patients to choose their meals was one of the ways through which healthcare professionals could empower patients to improve their meal-ordering experiences. They believed the initiative gave them control over a process they would otherwise have no authority to manage. This action signifies a creative way of enriching the in-patient experience by enabling them to order meals whenever they want and have them expeditiously delivered to their respective wards, subject to their individual needs and requirements.
De Kok et al. (2023) similarly used interviews to understand nurse leadership processes in a Dutch-based medical facility. They conducted 17 interviews and found that some healthcare institutions and their administrators have encountered a range of cross-functional issues when implementing new systems in the healthcare setting. Particularly, they believed that time and space issues influencing hospital care have dominated discussions surrounding the improvement of patient care. In this regard, the scholars cited practical, methodological, and ethical issues in the nursing practice as impediments to adopting new systems for improving patient care. As highlighted by the evidence gathered in the current probe, many healthcare institutions are modernizing their meal plans. In a book authored by Smith (2019), it was argued that, beyond seeking the cost-effective benefits of adopting change, healthcare institutions are looking to improve patients’ experiences and quality of care with this transition.
The main strength of the findings sampled in this project is their adherence to strict ethical requirements for managing information from human participants. These ethical considerations were observed during the collection of primary data related to the anonymity and confidentiality of the respondents’ views and their voluntary participation in the probe. These areas of ethical practice are commonly cited as areas of considerable interest in empirical research (Rich & Butts, 2021).
Comparatively, the small sample sizes reported in most of the cases were the source of their weakness because it means that their findings could not be generalized. Notably, most of the articles sampled in this study rarely used surveys because interviews were the preferred mode of data collection. This outcome was largely because they were qualitative investigations. The rampant use of the interview technique explains why most of the studies had a small sample of respondents, which could weaken their ability to develop reliable findings. Nonetheless, given that all publications were published within the last five years, it could be argued that their main strength is that their findings had a high validity because they were applicable to the contemporary healthcare setting.
Overall, understanding the challenges of developing effective meal-ordering plans has been critical in reviewing the quality of meal-ordering platforms and healthcare practices. Studies sampled in this review have highlighted multiple factors that are likely to affect this experience, including the level of professionalism of healthcare staff, availability of family support, and the integration of advanced hospital services. The modernization of patient care experiences through meal planning belongs to the last category of factors affecting patient experiences in the healthcare setting. Therefore, the overriding principle developed from the assessment of patient experiences in this framework of analysis is the idea that hospitals can significantly improve the quality of patient services by understanding individual patient journeys. This principle underscores the need to address individual healthcare needs in nursing. Particularly, it explains the importance of discussing the needs of patients with sensory or cognitive impairment needs.
Discussion of the Artefact
As highlighted above, using software application tools for meal planning is a relatively new area of healthcare research. Evidence suggests that meal-ordering apps are increasingly being adopted in the healthcare field, consistent with the upscaled use of IT-backed solutions to address healthcare challenges (Marangoni et al., 2019). The artifact proposed in the current probe was developed within this utility framework. However, it contains unique characteristics that distinguish it from other meal-ordering apps currently in use.
The distinguishing feature of this app is the use of individual imaging tools to improve and complete the meal ordering process. This feature sets it apart from current software application tools used in the same service because it is intended to appeal to groups of patients with unique cognitive and sensory challenges (Paoli et al., 2019). The integration of visual features in the app allowed patients to select their meals by clicking on images portrayed on electronic devices (Howarth et al., 2018). In the “All recipes” section of the App (see Appendix 1), the images for select meal choices are provided as the guiding selection methodology for the meal plans. It enabled patients to visually select the meal choices they want without necessarily relying on the written sections of the meal choices, which may be difficult to read or comprehend for those with reading impairments.
As highlighted in Appendix 1, the meal preferences of the proposed app were moderated depending on the nutritional needs of patients. The meal preferences of the patients were sorted into weekly and specialty food items for patients with unique nutritional requirements (Di et al., 2018). According to the meal planner identified in Appendix 1, the app has a provision for patients to develop a meal plan for every week. The meal design for two weeks is highlighted in the selection. Broadly, the meal plans were developed with image integration as the app’s unique feature.
In this artifact, daily and monthly meal plans could similarly be developed, but they are inconsistent with the meal ordering systems of most healthcare facilities. At the same time, the hospital’s meal plan caters to the needs of thousands of patients, thereby making a daily meal schedule too hectic for the hospital staff to implement (Facer-Childs et al., 2019). Therefore, it was difficult to integrate it within the hospital’s meal management model. Comparatively, the weekly meal plan made purchasing selected products possible and developing a meal preparation plan in advance (Muscaritoli, 2021). Therefore, the weekly meal plan formed a compromised meal planning system for the current artifact.
Business Case
Executive Summary
This business case is relevant to the development of a software application tool known as “Smart Diet.” Based on its application in telehealth interventions and eating disorder management, it is designed to address the meal-ordering needs of patients with cognitive and sensory disorders in the hospital ward setting (Zhou et al., 2022). Data would be gathered using primary and secondary research and analyzed using a software application tool known as Paprika (Hindsight Labs LLC. (2022). The instrument is crucial in developing an artifact that utilizes visual imagery features to enhance the meal ordering process for special needs patients.
The present project outlines the advantages and possible drawbacks of employing the new software in the healthcare setting, including cost implications and benefits to patients. The effects of the findings of this analysis on the nursing field are rooted in the quest to use automated meal-ordering systems to enhance care, especially for those with special needs.
Background
The needs of patients with specialized needs are often neglected in conventional medical practice (Zhou et al., 2022). This effect is poignant in adopting new technologies because most mainstream technologies appeal to general patient populations. Subject to this focus, patients with special needs have been excluded from many technological developments in the healthcare sector, including advancements made in improving the efficacy of meal-ordering systems (Rodríguez et al., 2018).
Subject to this observation, about 15% of people worldwide have special needs (United Nations, 2022). This figure translates to a similar number of patients who require support. The Smart Diet software is designed to address this problem by supporting the adoption of a new healthcare meal delivery model designed to appeal to the needs of patients with special meal ordering requirements. In this regard, it is determined that the new software would help patients with sensory and cognitive abilities to order their meals.
If unchecked, the burden of catering for patients with special needs may strain healthcare systems (NHS England, 2022b). Furthermore, the failure to address the needs of all patient groups may lead to increased cases of dissatisfaction (Wellings et al., 2022). Adopting the Smart Diet technology would help reduce patient dissatisfaction levels by offering relief through customized software that addresses unique patient needs.
The adoption of the new software App is likely to have a national effect by increasing the level of effectiveness in care delivery services because the current needs of special populations are not effectively addressed. At the same time, this business case is likely to affect local populations by allowing families and organizations to supplement patient care by providing customized solutions to improve meal-ordering processes (Wellings et al., 2022). In both situations, the software App is likely to have a positive impact on communities.
Current Service Provision
Historically, healthcare facilities have used a paper-based approach for meal-ordering data collection and preparing patient meals in the wards. The proposed Smart Diet software is designed to allow for the daily collection of such data prior to their use for better storage and management. In the current system, meal ordering systems are manually processed (Haraguchi et al., 2018). Specialized orders are similarly physically processed and set aside for a chef to prepare meals. Such an approach takes approximately two hours before meals are delivered to patients.
Again, this system is ineffective because, in some cases, the old paper-based system fails to impact patients. After all, they have been discharged or transferred to a different ward. This inefficient system could result in system malfunctions and patient disaffection (Ishibashi & Shimura, 2020). Therefore, improving the current meal-ordering model is necessary to make it more inclusive for all.
Proposal for Service and Financial Projections
The current project was developed using a software application known as Paprika. A free version application of the app was used to undertake the meal design process because of its flexibility and customization features (Hindsight Labs LLC, 2022). The feature equally has many benefits for software developers because it has no built-in dietary features that could undermine the design plan (Garaulet et al., 2022).
The recipe data collection was first completed before building a recipe database. Recipes were included directly from the respondents’ findings. When organizing saved recipes, meal categories were named to represent different categories of meals for the target patients (Hindsight Labs LLC, 2022). For example, those who suffered from dementia had their own meal plans, while those who suffered from stroke had their own setup that fit their unique needs and nutritional needs.
Given that the recipes and notes were amendable, including individual patient nutritional needs in the healthcare plan was possible. The software app enabled the researcher to scale ingredients up or down depending on nutritional requirements and the number of patients to be served (Wang et al., 2022). The software also included a feature that could convert nutritional measurements from metric to imperial scales. At the same time, it comprised weekly and monthly development plans for each patient group (see Appendix 1).
The proposed business plan is designed to increase the effectiveness of the current meal-ordering service model. It will modernize this system by automating the meal-ordering model and making it interactive for patients with special needs. Adopting new software in the nursing practice will likely come with additional costs (Ruiz-Mallorquí et al., 2021). Therefore, it is important to understand the financial implications of adopting the Smart Diet app to appreciate its viability as an entrepreneurial solution for improving meal-ordering systems (Blundel et al., 2018).
Similar to all other cost considerations of adopting new software applications in the healthcare environment, the financial implications of embracing new meal planning models should be among the top priorities for healthcare institutions (Gualandi et al., 2019). This statement is based on the confirmed ramifications of tolerating poor financial decisions with far-reaching effects on other aspects of the software development process.
Subject to an overview of key financial constraints affecting healthcare institutions, software development methodology, personnel costs, and training programs are likely to be the main cost implications for the new software management system. A trial scheme for implementing the new software is presented using the following financial projections in Table 4.1 below.
Table 4.1 Financial Projections for Smart Diet App (Source: Developed by Author of this Report).
In this pilot study, the projected cost saving amounts to £ 400,000 a year for the 100000-patient cohort. Coupled with efficacy gains likely to be realized from its implementation, healthcare institutions should consider adopting a Smart Diet for its wholesome benefits, such as augmented data management systems and improved service delivery (Ruiz-Mallorquí et al., 2021). To compute finances associated with the adoption of the new system, it is important to determine the initial software development costs and training expenses, as highlighted in Table 4.1 above. These two major investment areas will require an initial capital injection of £50,000. An additional expense of £15,000 will be attributed to staff costs.
In contrast, travel expenses will be the lowest expense incurred from the complete list of overheads attributed to the project via software testing plans. As highlighted in Table 4.1 above, this cost will be estimated to be £2,000. Cumulatively, it is expected that the above list of expenses will require an initial capital injection of £50,000 to cover all areas of spend. This amount of money is expected to be sourced from a business loan obtained from a local bank.
Drivers for New Service/Sustaining the Service
Like many healthcare projects adopted in healthcare settings, the proposed implementation plan for the Smart Diet software will naturally have key features that will enhance its adoption. In the context of the present discussion, these drivers will be assessed in terms of their contribution to national and local service provision objectives. Nationally, it will add to accommodating diversity in the patient setting (Yip & Cheon, 2020). This area of research comes from emerging concerns that the current healthcare methodology does not address the needs of all stakeholder groups, especially those with mental health challenges (Muscaritoli, 2021). Therefore, adopting the Smart Diet software would help enhance diversity and accommodate different patient groups.
Locally, the adoption of the Smart Diet software would mark a positive step towards identifying domestic solutions for improving meal ordering processes. At the same time, the healthcare community may take pride in developing customized solutions to address unique industry needs (Dolezel & McLeod, 2019). Nonetheless, patients are likely to be the biggest beneficiaries because the app would be addressing their unique needs and requirements. The app’s visual imaging features will help them communicate their preferred meals or services, thereby improving patient satisfaction (Su et al., 2020).
At an organizational level, the adoption of the new meal-ordering plan would play a critical role in boosting the image of the organization as an innovative medical center. The proposed training plan for adoption in the organization would equally help to improve the capacity of the nursing care staff to manage new technologies or address unique patient needs. Therefore, they are likely to benefit from increased levels of engagement in the meal-ordering process (Sabbah et al., 2020). Thus, this plan is likely to increase engagement between patients and their healthcare service providers.
Deliverable Outcomes/Activity to Date
The Smart Diet app trial proposed above can serve close to 100,000 meals to patients across 30 wards. Tentatively, the program is expected to serve a minimum of 90,000 patients and a maximum of 110,000 people. At the same time, traditionally, healthcare facilities have been using a paper-based model to collect meal-ordering data a day prior to their delivery.
In this system, completed cards are manually processed in the kitchen before service delivery (Alkhulaifi & Darkoh, 2022). Similar manual processing is used for specialized orders, which are then reserved for a chef to create meals. This paper-based system takes a lengthy lead time, but the new Smart Diet App is projected to cut this time by 50%. This estimation is made by computing the standard time taken by similar service providers when planning their meal ordering frequencies (Hayes et al., 2021). Nonetheless, automation would equally free up nursing personnel to cover other aspects of care delivery and not dwell a lot on meal processing.
Patient and Carer Feedback
The adoption of new software systems in healthcare settings is not a new phenomenon. However, the embracing of a new meal-ordering system is a novel idea (Talora, 2022). Its roots are firmly in evidence collected from other healthcare settings. This statement shows the success of automation in nursing processes. Deloitte Insights (2019) says the implementation of an Indian case project, namely Reducing Maternal and Newborn Deaths (ReMiND), affirms this fact. The program involved the development of software features that would allow patients in rural India to gain access to healthcare services and improve their well-being. It was designed to appeal to expectant mothers and the vulnerable population.
When Deloitte Insights (2019) developed the software, they took into account the ability of low-skilled workers to use the technology. Similarly, they ensured the app could be launched on a mobile phone, which increased its adoption rate. Xiao et al. (2019) believe that the source of success for such projects is the effective adoption of change management. Furthermore, they argue that the concept helps all stakeholders to understand their duties and responsibilities in the implementation plan (Xiao et al., 2019).
In this case study, the adoption of new digital tools to improve meal-ordering systems will help generate insights about users. The outcomes will be relevant in developing an effective implementation approach based on behavior change outcomes. The success of this project provides an example of how a simple care delivery App, such as the Reducing Maternal and Newborn Deaths (ReMiND) program, could help to save lives and improve healthcare costs. The same level of success is expected with the launch of the Smart Diet App.
Merits of the App
As highlighted in this document, the Smart Diet App proposed in this study is meant to improve the quality of patient care services. The primary areas of value created by the app include educational value, waste reduction, minimized risk of implementation, and order independence. These issues are discussed below.
Minimized Risk of Implementation
Visual imagery tools in software applications are a common phenomenon in nursing, induce feelings of relaxation, and are non-invasive (Permaul, 2020). It traces its roots to psychopathology and the study of medicine in ancient civilizations (Yip & Cheon, 2020). Research done in the second half of the 20th century demonstrated that the use of visual imagery tools reduces anxiety and pain among patients (Williamson et al., 2019). The quality of life and perceptions of wellness were similarly observed to emerge from these progressive areas of clinical practice (Godos et al., 2020).
Educational Value
The Smart Diet App has educational value because it enables healthcare service providers to track patients’ nutritional information and use the same data to improve their care plans (Haraguchi et al., 2018). This information is instrumental in understanding the nutritional intake proportions of specific food compounds, such as sodium, which may have health implications for select groups of patients (Tahara & Shibata, 2022). If the proportions are exceeded, a red flashlight will beam, indicating the need for remedial action. Based on the presence of these features in the app, nutritional balance is likely to be maintained in such a setup.
Waste Reduction
Waste reduction is another important benefit of adopting the new software in the hospital meal ordering system. This benefit may be important through efficient meal ordering and serving processes (Currenti et al., 2021). The goal is to streamline the meal ordering system to minimize opportunities for wastage because it would minimize the quantities of leftover food (Chang et al., 2021). Standardized meal sets would make it possible to prepare food more efficiently than is currently the case in most healthcare facilities because meeting individual meal requirements is costly and time-consuming.
Order Independence
Convenience and safety are some of the primary benefits of adopting the proposed app. However, order independence is an additional feature that would likely be enjoyed by the patients and their family members because the Smart Diet App will give users the autonomy to manage their meal ordering processes effectively (Godos et al., 2021). At the same time, hospitals can control diets through their centralized data management repositories, thereby enabling the independent meal ordering process to be synchronized with a hospital’s meal ordering plan.
Risks Associated with the App
Similar to challenges associated with the adoption of new systems, the Smart Diet application is naturally likely to be resisted by some nurses because of their unfamiliarity with the tool. In this regard, several risks could be associated with the development of the proposed artifact. They include the risk of ordering wrong meals due to similarities in images and the risk of system malfunction (Mathew et al., 2020). This challenge could emerge from the reliance on visual imaging techniques as the basis for making meal orders (Chaix et al., 2019). Thus, it should be expected that patients might confuse one meal plan with another based on similarities in images.
Discussion
Change Management
As highlighted in this paper, the process of adopting new systems in the healthcare sector is complex. Concisely, several factors have to be reviewed for the successful adoption of new systems. For example, nursing theories on innovation have been used to discuss the implications of employing innovative tools in the healthcare setting (Sensmeier, 2021). They have discussed adoption rates of innovation tools in the nursing practice (de Kok et al., 2023).
For instance, some scholars have used existing theories to explain implementation rates of new patient management systems (Mohammadi et al., 2018). Furthermore, de Kok et al. (2023) (and Shimizu et al. (2018) believe that the motivation for other researchers to develop theories and leadership models in nursing is to explain circumstances that moderate the process of accepting and integrating new systems in the healthcare setting. Broadly, their inputs have provided contextual support for transformational change in the healthcare setting.
Based on the theoretical background of analysis highlighted above, the change management process can be explained using several associate theories, including Lewin’s (1940) change management model and the Plan-Do-Study-Act (PDSA) developed by Walter Stewart in the 1920s. PDSA has four key stages, which include developing a plan, implementing it, studying it, and adopting or rejecting proposed changes (Christoff, 2018). As seen from these steps, this model elaborates on the explanation of change management processes. However, it was not used in the current project because it contains a mixed-up order of execution and is unable to use the model repetitively.
The diffusion of innovation theory could be used to explain the above phenomenon. This model is unique from others mentioned in this paper because, unlike processes that lead to its adoption, it focuses on discussing the characters of patients as innovation receptors (Frei-Landau et al., 2022). The theory suggests that the success of innovation adoption is dependent on people’s attitudes towards it. Similarly, it describes interphase transitions between different stages of innovation adoption based on the characteristics of the target group. Subject to this framework of review, five key groups of people influence the rate of innovation adoption in organizations. They include innovators, early adopters, early majority, late majority, and laggards, as highlighted in Figure 2.1 below.
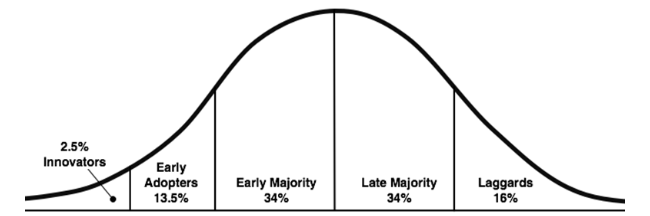
As highlighted in Figure 2.1 above, innovators from the first group of institutions are likely to embrace new ideas during the introduction phase of a new idea. They often take the original risk of experimenting with new technologies before other healthcare facilities participate in the same process. “Early adopters” comprise the second group of users, and they often accept new technologies after prolonged use by other healthcare institutions (Gereffi & Bamber, 2018). It is believed that this group of people forms about 13.5% of users in the innovation lifecycle.
The smallest group of organizations to adopt new technology is ‘laggards,’, and their involvement is based on the confirmed successes of technology adoption in other organizations. Therefore, it can be assumed that this population group is the most risk-averse of all the users described above. Based on the above categorization of innovation adopters, the introduction of the Smart Diet app in the hospital environment is likely to undergo the five stages of the option defined above. Overall, the above model shows that researchers have examined the transformation of change practices through systems and process analyses by describing how new systems and models in the nursing practice are implemented and adopted.
Comparatively, Lewin’s (1940) change theory has explored processes involved in idea dissemination (Lang & Nash, 2023). The three-stage model suggests that change management processes are completed through the evolution of three stages of transformation: unfreezing, change/moving, and refreezing, as highlighted in Figure 5.1 below.
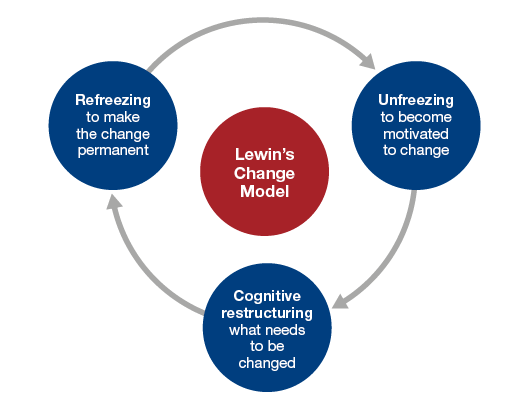
Figure 5.1 above shows that change management processes can be achieved when organizations transition through the “unfreezing,” “moving,” and “refreezing” stages. As explained in nursing leadership literature, transitions across these three stages of development require flexibility in values and attitudes (Taylor &Webster-Henderson, 2016). Attitudes and behaviors that lead to the generation of disconfirming data are similarly analyzed in this assessment stage. The intention is to reduce them to create adequate room for the adoption of new ideas (Zonneveld et al., 2021).
Overall, these theoretical constructs have been useful in predicting the success of technology integration in the healthcare sector. This change management framework was selected as the primary model for analysis because it is logical and simple to understand. Therefore, the implementation plan for its use is described in Table 5.1 below.
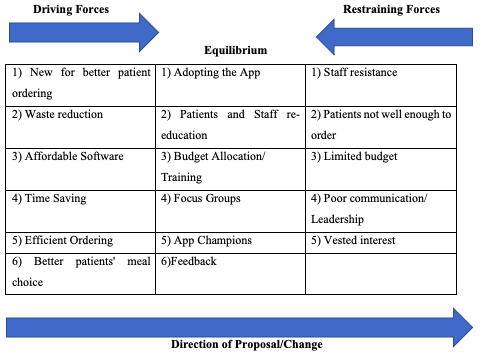
Unfreezing
Good leadership is essential at the unfreezing stage to effect change. The driving forces listed above are the requirement for better patient meal ordering, the requirement for waste reduction, the advent of affordable software, and the desire to save time on the wards and make better patient meal choices (Hayes et al., 2021). The Smart Diet app development above, which can provide around 100,000 meals to patients across 30 wards in three months, is described in the unfreezing stage. The traditional paper-based system takes a lead time of about two hours before the final product is delivered (Hayashida et al., 2021). Inefficient systems could result in financial losses due to poor resource use (Sittig et al., 2020).
The Smart Diet App will be introduced in the healthcare setting by making it available to patients’ mobile devices, such as a tablet or smartphones. Given that the system will be virtually operated, patients will have an opportunity to order meals through their phones as well. The goal is to ensure that the needs of all groups of patients are sufficiently served.
The proposed application will play a significant role in replacing outdated ordering systems (Ishibashi & Shimura, 2020). The traditional ordering model was ineffective in meeting these needs because the time for delivering the food was often unclear, thereby having a negative implication on patients’ experiences (Shimura et al., 2020). Nursing leadership can be viewed as a broken system leading to patient dissatisfaction (Marquis & Houston, 2015). However, the flexibility of the digital model proposed in the app gives patients choice.
Moving
Movement can be achieved by changing beliefs and attitudes and adjusting processes and systems that determine those behaviors (Hayes, 2014). Staff and patient training will be pivotal at this stage, as well as budget allocation to achieve traction. The use of ‘App’ champions as advocates to promote the use of the app on the wards will be useful at this stage of moving to a new paradigm.
The implementation process for the proposed software application will be administered in compliance with hospital policies. It will be introduced into the existing system as an improvement to the process as opposed to a “re-invention of the wheel” (Diener et al., 2020). In this regard, the meal ordering system should be integrated with an institution’s Electronic Patient Record (EPR) to compare and contrast previous meal plans with individual nutritional requirements (Tahara et al., 2021). However, this action should be done within the legal and moral limits governing the management of patient data (Dolezel & McLeod, 2019).
To further improve the effectiveness of the new model, the new meal ordering system may be synchronized with a hospital’s bed management system to account for changes to patients’ positioning within wards during meal delivery (Currenti et al., 2020). These integrative models will allow nurses to cancel or modify orders as the case may be. In this system, the order placement system will notify the kitchen staff whenever a new order is placed (Martins et al., 2021). New tallies on the display screens will be generated in the process, thereby leading to information accuracy (Charlot et al., 2021). Therefore, chefs will rely on the live status of orders to fulfill patients’ requirements.
Refreezing
This stage involves reinforcing new ways of operating to avoid going back to the old ways (Hayes, 2014), and positive reinforcement and incentives can embed these new ways of conduct.
Hospitals are leveraging technological tools to achieve the above-mentioned change management objectives. Nursing leadership qualities have largely moderated their success (Harne-Britner, 2022). Stemming from the quest to modernize existing patient care systems, meal-ordering experiences will be policed to optimize patients’ experiences in a healthcare facility.
To implement this plan, a color-coding feature will be introduced in Smart Diet to distinguish meal plans. Similarly, each visual image will be paired with digitized texts to ensure the details are consistent across all platforms of service delivery (Permaul, 2020). A rigorous training program will further support this plan, and nurses will be required to confirm their meal requests with the patients before they are prepared. This strategy will be introduced as a standard protocol or procedure for accepting meal requests.
At the same time, from a technical perspective, each meal-ordering request will be subjected to a confirmatory interface page that will require patients to confirm whether the selected visual image represents the textual description of the same meal before order completion. Discrepancies can be corrected at this stage of assessment because the software feature will have an “edit” option button that would allow patients to edit their orders before completing their requests. Thus, it will be possible for the new software to update itself based on its implementation history (McPherson et al., 2020). As part of the risk planning process, the implementation plan will be audited periodically based on an assessment of key features of the audit cycle described in Figure 5.2 below.
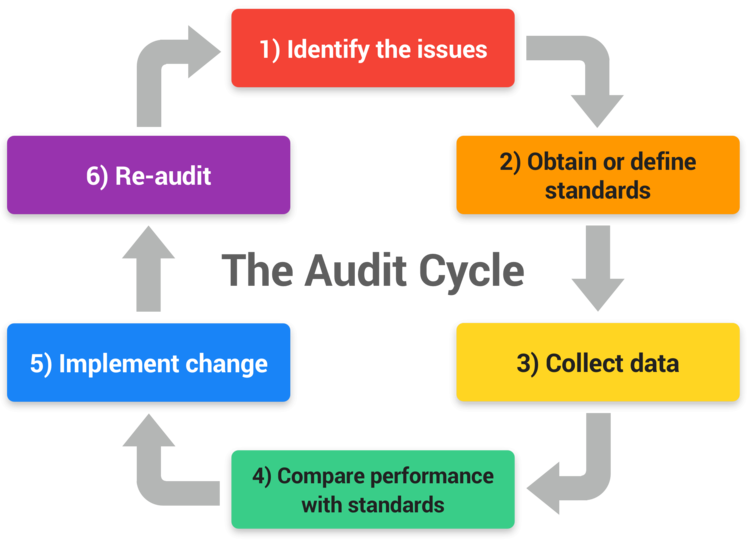
Broadly, the audit cycle highlighted in Figure 5.2 above will be instrumental in ensuring the Smart Diet App project achieves its intended milestones. Key steps involved will be centered on identifying key issues, defining standards, collecting data, implementing change, and re-auditing processes to realize optimum results. The East Midlands Emergency Medicine Educational Center (2018) has used this audit cycle to undertake clinical audits. It is selected for use in the current case study because of its appropriateness in implementing digital service systems (Mathew et al., 2020). The audit plan will include regular reviews and assess the organization’s performance relative to its strengths and weaknesses.
Leadership Plan
My leadership style for the adoption of the app will span from a democratic to transformational style as I seek to enable cooperation from patients and staff. In my leadership role, I will fulfill the requirements listed in Table 5.2 below.
Table 5.2 Seven Tasks that Leader Needs (Hayes, 2014).
Adopting the Smart Diet App requires effective leadership, which is an important feature for the successful adoption of the current artifact. It is part of the wider change management strategy aimed at completing transformational change processes in the nursing practice (Hiat & Creasy, 2016). Nursing leadership styles vary based on the characteristics and behaviors of those who have been entrusted to spearhead the change management process.
To this end, researchers have mentioned several leadership styles as appropriate frameworks for guiding the change management process in the healthcare setting (NorthHouse, 2019; Western, 2019). They include the democratic, transformational, autocratic, and servant leadership styles (Faraz et al., 2023). The democratic leadership style is synonymous with high levels of participation across different cadres of engagement (Sabbah et al., 2020).
Comparatively, the autocratic leadership style involves minimal engagement with stakeholders in decision-making and can result in resistance and apathy. At the same time, the servant leadership style is people-based and encourages healthcare staff to work for the benefit of patients as their primary responsibility. The transformational leadership style is disruptive because it uses creativity and emotional intelligence to complete operational functions (Faraz et al., 2023). In this regard, it is unpredictable, unlike other leadership styles described above.
Subject to the characteristics of the leadership styles highlighted above, the Transformational framework emerges as the most preferable style for implementing the above-mentioned changes (Hayes et al., 2021). This selection is informed by the transformational nature of the project as it seeks to introduce a new meal order system and eliminate the existing one (Smith, 2019). Transformational leadership is preferable because its leaders are influential people who can inspire change by convincing employees to abandon old systems and embrace new ones by justifying why they should make such a change (Zonneveld et al., 2021). Therefore, persuasion is the currency of this leadership style, and it has been associated with high rates of commitment and employee performance in the nursing field (Sabbah et al., 2020). Therefore, it is the most appropriate leadership style to adopt in the current project.
The above-mentioned transformation plan offers immense benefits to hospital staff and patients alike. Indeed, studies have shown that healthcare facilities that have embraced such transformative plans have registered increased worker productivity. Based on these benefits, independent healthcare organizations and institutions have advocated for the widespread adoption of automated meal-ordering solutions (Taylor et al., 2016). Nationally, researchers have linked the poor services of integrated systems providers with the use of manual operating systems (Talorav, 2022). Faraz et al. (2023) suggested that hospital administrators seek digital solutions to improve the efficacy of their operations.
Conclusion
As highlighted in this project, the proposed Smart Diet App is likely to include several features that would support the meal-ordering process for groups of patients with special needs and make it more efficient. The literature review section of this paper showed a research gap that was characterized by the lack of empirical findings on the development of automated meal-ordering technology for patients with cognitive and sensory impairments. There is a great need for quantitative research to establish the efficacy of software and meal-ordering Apps in improving patient outcomes. The artifact developed is a product of an evidence-based examination of improvements that could be adopted to improve in-patient experiences inwards.
The proposed Smart Diet App has been designed to achieve several objectives, including saving time for people or organizations that need to develop meal plans for patients with sensory and cognitive impairments. Similar Apps have been developed to help people who intend to serve many people with unique nutritional needs. This feature matches the characteristics of the present study because of the researcher’s meal plan setting. The financial implications of developing the proposed meal ordering system support its adoption because the new model would be cheaper to maintain than the current generalized meal ordering system.
These cost advantages are further supported by efficiency gains that are likely to be realized if patients have an opportunity to customize their meal plans. In this regard, the Smart Diet application tool will enable users to select specific meal-ordering plans and recipes. This development has many benefits for patients and nurses alike because it helps healthcare providers track patients’ dietary information for improved service delivery. The app can be made available to patients because it can be administered using a mobile device. Therefore, the software features integrated into the proposed software application will help them order the foods they want using visual imaging tools.
Future modifications can be included in the application to enable guests to make similar orders and pay for them via the app. The ability to track the order from the meal ordering stage of the delivery process can equally be included in the system to make it more responsive and interactive. This feature would enable patients to be considerate about their meal ordering process and give healthcare practitioners data that would be useful in improving response times. This strategy can help them to enhance their sense of control over the meal ordering process.
Subject to the importance of including patients’ experiences and the inputs of healthcare professionals in the software development process, it is important to embrace the co-creation of data for both sets of participants. This technique will give a broad-based outlook of the implications of software adoption in the meal ordering process. These tools will allow users to self-monitor and self-manage their meal plans. This recommendation emerges from the successful use of digital tools in addressing the mental healthcare needs of patients in the healthcare setting. They highlight the importance of using both theory and data-driven techniques in developing software application tools for future practice.
Overall, the findings of this study are limited to their indicative nature. However, operational and contextual issues may be considered for review if they are to be adopted or employed in a specific healthcare system due to policy consistencies. The second limitation of this study relates to the target population, which is largely comprised of people with reading or cognitive disabilities. Therefore, the findings of the study will be limited in application to these groups of patients.
References
Abhari, S., Jalali, A., & Jaafar, M. (2022). Determinants influencing customers’ acceptance of smart restaurants in Penang, Malaysia. Arab Gulf Journal of Scientific Research, 40(3): 264-279. Web.
Alkhulaifi, F., & Darkoh, C. (2022). Meal timing, meal frequency and metabolic syndrome. Nutrients, 14(9), 1719. Web.
Blundel, R., Lockett, N., & Wang, C. (2018). Exploring entrepreneurship. Sage Publications.
Boehm, L. M., Jones, A. C., Selim, A. A., Virdun, C., Garrard, C. F., Walden, R. L., Wesley, E., & Hosie, A. (2021). Delirium-related distress in the ICU: A qualitative meta-synthesis of patient and family perspectives and experiences. International Journal of Nursing Studies, 5(2): 122-129. Web.
Boston University School of Public Health. (2022). Diffusion of innovation theory. Web.
Bucci, S., Schwannauer, M., & Berry N. (2019). The digital revolution and its impact on mental health care. Psychology and Psychotherapy, 92(2): 277-297. Web.
Chaix, A., Manoogian, E.N.C., Melkani, G.C., & Panda, S. (2019). Time-restricted eating to prevent and manage chronic metabolic diseases. Annual Review and Nutrition, 3(9): 291–315. Web.
Chang, Z. S., Boolani, A., Conroy, D.A., Dunietz, T., & Jansen, E.C. (2021). Skipping breakfast and mood: The role of sleep. Nutrition and Health, 7(2), 1-7. Web.
Charlot, A., Hutt, F., Sabatier, E., & Zoll, J. (2021). Beneficial effects of early time-restricted feeding on metabolic diseases: Importance of aligning food habits with the circadian clock. Nutrients, 1(3):1-14. Web.
Christoff, P. (2018). Running PDSA cycles. Current Problems in Pediatric and Adolescent Health Care, 48(8), 198–201. Web.
Currenti, W., Godos, J., Castellano, S., Caruso, G., Ferri, R., Caraci, F., Grosso, G., & Galvano, F. (2021). Association between time restricted feeding and cognitive status in older Italian adults. Nutrients, 1(3) :191. Web.
Currenti, W., Godos, J., Castellano, S., Mogavero, M.P., Ferri, R., Caraci, F., Grosso, G., & Galvano, F. (2020). Time restricted feeding and mental health: A review of possible mechanisms on affective and cognitive disorders. International Journal of Food Science and Nutrition, 7(2):1–11. Web.
Davis, R., Rogers, M., Coates, A. M., Leung, G., & Bonham, M. P. (2022). The impact of meal timing on risk of weight gain and development of obesity: A review of the current evidence and opportunities for dietary intervention. Current Diabetes Reports, 22(4): 147-155. Web.
De Kok, E., Janssen-Beentjes, K., Lalleman, P., Schoonhoven, L., & Weggelaar, A. M. (2023). Nurse leadership development: A qualitative study of the Dutch excellent care program. Journal of Nursing Management, 2(3): 1-11. Web.
Deloitte Insights. (2019). Digital health technology. Web.
Diener, E., Emmons, R.A., Larsen, R.J., Griffin, S. (2020). The satisfaction with life scale. Journal of Personal Assessment, 4(9): 71–75. Web.
Di Francesco, A., Di Germanio, C., Bernier, M., & de Cabo, R. (2018). A time to fast. Science, 362(6416), 770–775. Web.
Diamond, T., Cameron, F., & Bequette, B. W. (2022). A new meal absorption model for artificial pancreas systems. Journal of Diabetes Science and Technology, 16(1): 40-51. Web.
Dolezel, D., & McLeod, A. (2019). Big data analytics in healthcare: Investigating the diffusion of innovation. Perspectives in Health Information Management, 1(16), 1-11.
East Midlands Emergency Medicine Educational Center. (2018). Clinical audit. Web.
Facer-Childs, E.R., Middleton, B., Skene, D.J., & Bagshaw, A.P. (2019). Resetting the late timing of “night owls” has a positive impact on mental health and performance. Sleep Medicine, 3(6): 236–247. Web.
Faraz, N. A., Xiong, Z, Mehmood, S. A., Ahmed, F., & Pervaiz, K. (2023). How does servant? leadership nurture nurses, job embeddedness? Uncovering sequential mediation of psychological contract fulfillment and psychological ownership. Journal of Nursing Management, 2(2), 1-11. Web.
Frei-Landau, R., Muchnik-Rozanov, Y., Avidov-Ungar, O. (2022). Using Rogers’ diffusion of innovation theory to conceptualize the mobile-learning adoption process in teacher education in the COVID-19 era. Education Information Technology, 27(9): 12811-12838. Web.
Garaulet, M., Gómez-Abellán P., Alburquerque-Béjar J. J., Lee Y. C., Ordovás J. M., & Scheer F.A. (2022). Timing of food intake predicts weight loss effectiveness. International Journal of Obesity, 3(7): 604–611. Web.
Gereffi, G., & Bamber, P. (2018). Global firms in the medical devices industry. Web.
Godos, J., Currenti, W., Angelino, D., Mena, P., Castellano, S., Caraci, F., & Galvano, F., (2020). Diet and mental health: review of the recent updates on molecular mechanisms. Antioxidants, 9(1): 346. Web.
Godos, J., Grosso, G., Castellano, S., Galvano, F., Caraci, F., & Ferri, R. (2021). Association between diet and sleep quality: A systematic review. Sleep Medical Review, 5(7): 101-120. Web.
Gualandi, R., Masella, C., Viglione, D., & Tartaglini, D. (2019). Exploring the hospital patient journey: What does the patient experience? PLoS One, 14(12): 1-12. Web.
Haraguchi, A., Fukuzawa, M., Iwami, S., Nishimura, Y., Motohashi, H., Tahara, Y., & Shibata, S. (2018). Night eating model shows time-specific depression-like behavior in the forced swimming test. Science Review, 8(2):10-18. Web.
Harne-Britner, S. (2022). Visionary leader award 2021 visionary leader runner-up carol grove. Nursing Management, 53(4): 38-40. Web.
Harvey, M., & Land, L (2016). Research methods for nurses and midwives. London: Sage Publications.
Hayashida, T., Shimura, A., Higashiyama, M., Fujimura, Y., Ono, K., Inoue, T. (2021). Psychosomatic stress responses and sleep disturbance mediate the effects of irregular mealtimes on presenteeism. Neuropsychiatry Disorder Treatment, 1(7): 315–321. Web.
Hayes, J. F., Balantekin, K. N., Fitzsimmons-Craft, E. E., Jackson, J. J., Ridolfi, D. R., Boeger, H. S., Welch, R. R., & Wilfley, D. E. (2021). Greater average meal planning frequency predicts greater weight loss outcomes in a worksite-based behavioral weight loss program. Annals of behavioral medicine: a publication of the Society of Behavioral Medicine, 55(1), 14–23. Web.
Healthcare Information Systems and Management Society. (2020). Supporting the relationship between seniors and healthcare technology. Web.
Hiat, J, M., & Creasy, T. (2016). Change management: The people side of change. Prosci.
Hindsight Labs LLC. (2022). Paprika: Recipe manager. Web.
Howarth, A., Quesada, J., Silva, J., Judycki, S., & Mills, P.R. (2018). The impact of digital health interventions on health-related outcomes in the workplace: A systematic review. Digital Health, 4(2), 552-576. Web.
Ishibashi, Y., & Shimura, A. (2020). Association between work productivity and sleep health: A cross-sectional study in Japan. Sleep Health, 6(1): 270–276. Web.
Koch, C.E., Begemann, K., Kiehn, J.T., Griewahn, L., Mauer, J., Hess, M.E., Moser, A., Schmid, S.M., Brüning, J.C., & Oster, H. (2020). Circadian regulation of hedonic appetite in mice by clocks in dopaminergic neurons of the VTA. National Communications, 1(1): 3071. Web.
Lang, K., & Nash, A. (2023). A new approach to building a strong leadership bench. Nursing Management, 54(3): 8-12. Web.
MacKenzie-Shalders, K., Maunder, K., So, D., Norris, R., & McCray, S. (2020). Impact of electronic bedside meal ordering systems on dietary intake, patient satisfaction, plate waste and costs: A systematic literature review. Nutrition and Dietetics, 77(1): 103-111. Web.
MacKinnon, G. E., & Brittain, E. L. (2020). Mobile health technologies in cardiopulmonary disease. Chest, 157(3): 654-664. Web.
Mansfield, E., Sandercock, J., Dowedoff, P., Martel, S., Marcinow, M., Shulman, R., Parks, S., Peters, M.-L., Versloot, J., Kerr, J., & Zenlea, I. (2021). Implementing integrated care pilot projects in hospital settings – An exploration of disruptive practices. Journal of Integrated Care, 29(2): 126-140. Web.
Marangoni, F., Martini, D., Scaglioni, S., Sculati, M., Donini, L. M., Leonardi, F., Agostoni, C., Castelnuovo, G., Ferrara, N., Ghiselli, A., Giampietro, M., Maffeis, C., Porrini, M., Barbi, B., & Poli, A. (2019). Snacking in nutrition and health. International Journal of Food Sciences and Nutrition, 70(8): 909–923. Web.
Marquis, B., & Houston, C. J. (2015). Leadership roles and management functions in nursing. London: Wolters Kluwer Health.
Martins, L.B., Braga, J.R., Sanches, M., Jacka, F., Berk, M., & Teixeira, A.L. (2021). Nutrition-based interventions for mood disorders. Expert Review and Neurotherapy, 2(1): 303–315. Web.
Mathew, G.M., Hale, L., & Chang, A.M. (2020). Social jetlag, eating behaviours and BMI among adolescents in the USA. British Journal of Nutrition, 1(2): 979–987. Web.
Mauch, C. E., Wycherley, T. P., Laws, R. A., Johnson, B. J., Bell, L. K., Golley, R.K. (2018). Mobile apps to support healthy family food provision: systematic assessment of popular, commercially available apps. JMIR Mhealth Uhealth, 6(12):118-167. Web.
McPherson, S., Wicks, C., & Tercelli, I. (2020). Patient experiences of psychological therapy for depression: A qualitative metasynthesis. BMC Psychiatry, 20(1): 313-317. Web.
Mohammadi, M. M., Poursaberi, R., & Salahshoor, M. R. (2018). Evaluating the adoption of evidence-based practice using Rogers’s diffusion of innovation theory: A model testing study. Health Promotion Perspectives, 78(1): 25-32. Web.
Muscaritoli, M. (2021). The impact of nutrients on mental health and well-being: Insights from the literature. Frontier Nutrition, 8(6), 56-69. Web.
NHS England. (2022a). Learning disability and autism: Our work. Web.
NHS England. (2022b). NHS long-term plan. Web.
NHS-UK. (2022). We lead the NHS in England to deliver high quality services for all. Web.
NIH Office of Communications. (2020). What are reading disorders? Web.
NorthHouse, P. G. (2019). Introduction to leadership (4th ed.). Lansing, MI: Sage Publications.
Odendaal, W. A., Anstey, J., Leon, N., Goudge, J., & Griffiths, F. (2020). Health workers’ perceptions and experiences of using m-health technologies to deliver primary healthcare services: A qualitative evidence synthesis. Cochrane Database of Systemic Reviews, 26(3): 119-129. Web.
Ogata, H., Hatamoto, Y., Goto, Y., Tajiri, E., Yoshimura, E., Kiyono, K., Uehara, Y., Kawanaka, K., Omi, N., & Tanaka, H. (2019). Association between breakfast skipping and postprandial hyperglycaemia after lunch in healthy young individuals. British Journal of Nutrition, 1(2): 431–440. Web.
Open Access Government. (2021). Implementing digital food menus in hospitals. Web.
Paoli, A., Tinsley, G., Bianco, A., & Moro, T. (2019). The influence of meal frequency and timing on health in humans: The role of fasting. Nutrients, 11(4), 719. Web.
Permaul, J. (2020). Using visualisation and imagery for health and wellbeing. Web.
Ribbink, M. E., Roozendaal, C. C., MacNeil-Vroomen, J. L., Franssen, R., & Buurman, B. M. (2021). Patient experience and satisfaction with admission to an acute geriatric community hospital in the Netherlands: A mixed method study. Journal of Integrated Care, 29(4): 390-402. Web.
Rich, K. L., & Butts, J. B. (2021). Philosophies and theories for advanced nursing practice. New York, NY: Jones & Bartlett Learning.
Rodríguez, N., Mourelle, M. R., Diéguez, M., & De, M. J. (2018). A study of the dental treatment needs of special patients: Cerebral paralysis and Down syndrome. European Journal of Pediatric Dentistry, 19(3), 233–238. Web.
Ruiz-Mallorquí, M. V., Aguiar-Díaz, I., González-López, V. B. (2021). Arrangements with the NHS for providing healthcare services: Do they improve financial performance of private for-profit hospitals in Spain? Health Economics Review, 10(1): 9-17. Web.
Sabbah, I. M., Ibrahim, T. T., Khamis, R. H., Bakhour, H. A., & Sabbah, S. M. (2020). The association of leadership styles and nurses’ well-being: A cross-sectional study in healthcare settings. Pan African Medical Journal, 24(36): 328-332. Web.
Sensmeier, J. (2021). Realizing nurse-led innovation. Nursing Management, 52(6): 14-19. Web.
Shimizu, H., Hanzawa, F., Kim, D., Sun, S., Laurent, T., Umeki, M., Ikeda, S., Mochizuki, S., & Oda, H. (2018). Delayed first active-phase meal, a breakfast-skipping model, led to increased body weight and shifted the circadian oscillation of the hepatic clock and lipid metabolism-related genes in rats fed a high-fat diet. PLoS ONE, 1(3): 1-9. Web.
Shimura, A., Sugiura, K., Inoue, M., Misaki, S., Tanimoto, Y., Oshima, A., Tanaka, T., Yokoi, K., & Inoue, T. (2020). Which sleep hygiene factors are important? Comprehensive assessment of lifestyle habits and job environment on sleep among office workers. Sleep Health, 6(1): 288–298. Web.
Shukla, A. P., Dickison, M., Coughlin, N., Karan, A., & Mauer, E. (2019). The impact of food order on postprandial glycaemic excursions in prediabetes. Diabetes Obesity and Metabolism, 21(2): 377-381. Web.
Sittig, D. F., Wright, A., Coiera, E., Magrabi, F., & Ratwani, R. (2020). Current challenges in health information technology-related patient safety. Health Informatics Journal, 26(1): 181-189. Web.
Smith, M. (2019). Nursing theories and nursing practice. New York, NY: Davis Company.
Su, J. H., Zheng, P., Kinrot, S. S, Bintu, B., & Zhuang, X. (2020). Genome-Scale Imaging of the 3D organisation and transcriptional activity of chromatin. Cell, 182(6):1641-1659. Web.
Tahara, Y., Makino, S., Suiko, T., Nagamori, Y., Iwai, T., Aono, M., & Shibata, S. (2021). Association between irregular meal timing and the mental health of Japanese workers. Nutrients, 13(8), 2775. Web.
Tahara, Y., & Shibata, S. (2022). Chronobiology and nutrition. Neuroscience, 2(5) : 78–88. Web.
Talora, J. (2022). Technology and innovation. Web.
Taylor, R., &Webster-Henderson, B. (2016). The essentials of nursing leadership. Lansing, MI: Sage.
UK Leadership Center. (2022). Lewin’s model of change. Web.
United Nations. (2022). Factsheet on persons with disabilities. Web.
Vela, E., Plaza, A., Carot-Sans, G., Contel, J. C., Salvat-Plana, M., Fabà, M., Giralt, A., Ribera, A., Santaeugènia, S., & Piera-Jiménez, J. (2022). Data and care integration for post-acute intensive care program of stroke patients: effectiveness assessment using a disease-matched comparator cohort. Journal of Integrated Care, 30(4), 434-455. Web.
Wang, X., Hu, Y., Qin, L. Q., & Dong, J. Y. (2022). Meal frequency and incidence of type 2 diabetes: A prospective study. The British Journal of Nutrition, 128(2), 273–278. Web.
Wellings, D., Jefferies, D., Maguire, D., & Appleby, J. (2022). Public satisfaction with the NHS and social care in 2021: Results from the British Social Attitudes survey. Web.
Western, S. (2019). Leadership, a critical text (3rd ed.). London: Sage Publications.
Williamson, A., Dellve, L., & Karltn, A. (2019). Nurses’ use of visual management in hospitals- A longitudinal, quantitative study on its implications on systems performance and working conditions. Journal of Advanced Nursing, 75(6): 1-12. Web.
Winsight LLC. (2023). What patients want: A better meal-ordering experience. Web.
Xiao, Q., Garaulet, M., & Scheer, F. A. J. L. (2019). Meal timing and obesity: Interactions with macronutrient intake and chronotype. International Journal of Obesity (2005), 43(9), 1701–1711. Web.
Yip, T., & Cheon, Y. M. (2020). Sleep, psychopathology and cultural diversity. Current Opinion in Psychology, 8(34):123-127. Web.
Yoon, G., Li, C., & Choi, J. J. (2022). ‘In search of time to bring the message on social media: Effects of temporal targeting and weather on digital consumers’, Frontier Psychology, 16(13), 1-11. Web.
Zerón-Rugerio, M. F., Hernáez, Á., Porras-Loaiza, A.P., Cambras, T., & Izquierdo-Pulido, M. (2019). Eating jet lag: A marker of the variability in meal timing and its association with body mass index. Nutrients, 1(1): 2980. Web.
Zhou, X., Bambling, M. and Edirippulige, S. (2022). A mixed-method systematic review of text-based tele-health interventions in eating disorder management. Journal of Health Research, 36(6): 1149-1165. Web.
Zonneveld, N., Pittens, C., & Minkman, M. (2021). Appropriate leadership in nursing home care: A narrative review. Leadership Health Services, 2(2): 16–36. Web.